DataRich Consulting Publications
Books
Analysis of clinical trial results is easier when the data is presented in a visual form. However, clinical graphs must conform to specific guidelines in order to satisfy regulatory agency requirements.
If you are a programmer working in the healthcare and life sciences industry and you want to create straightforward, visually appealing graphs using SAS, then this book is designed specifically for you.
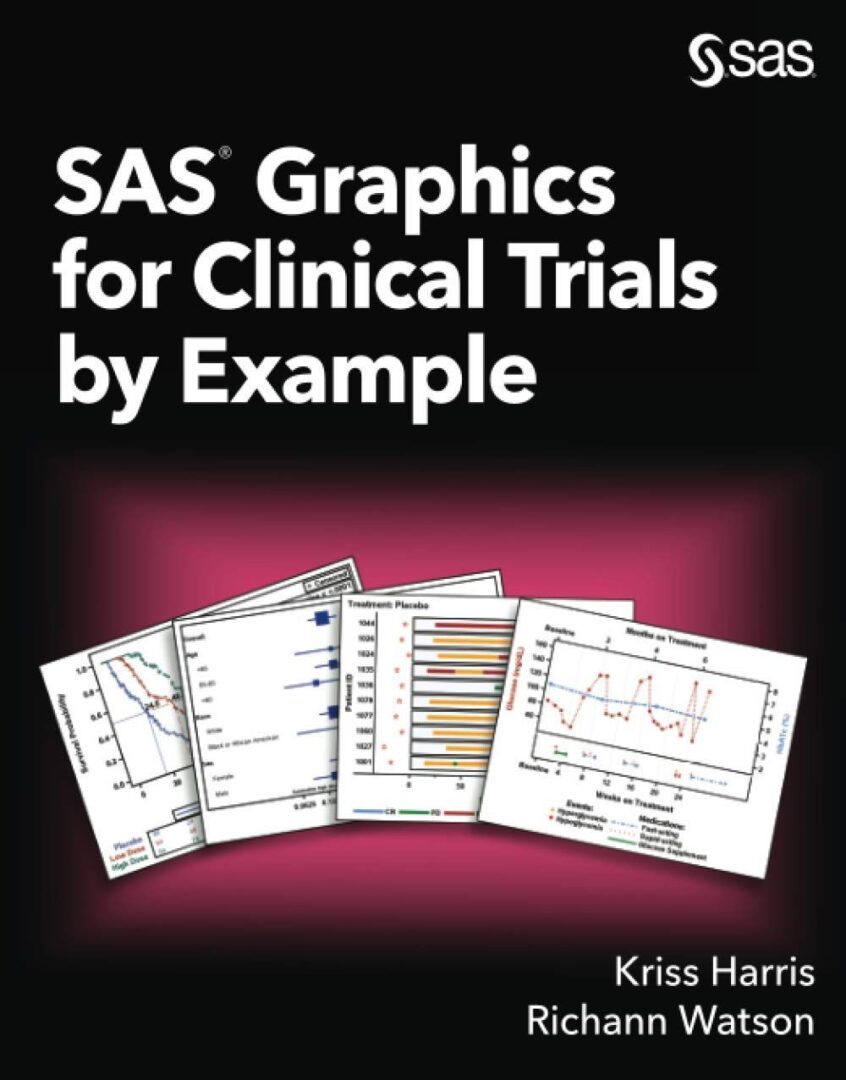
Buy the Book:
CDISC PAPERS
Richann Jean Watson; Karl Miller; Paul Slagle
There has always been confusion as when to use some of the various flags (ANLzzFL, CRITyFL) or category variables (AVALCATy) reserved for ADaM basic data structure (BDS). Although some of these variables can be used interchangeably it might help to come up with rules to help keep a consistency of when to use these variables. Furthermore, there may be some situations where the creation of a new data set would be the better solution. These data sets are referred to as parent-child data sets throughout the paper. This paper will focus on rules that the authors follow when developing ADaM data set specifications for the proper use of ANLzzFL, CRITy/CRITyFL, AVALCATy or when a parent-child data set option is more feasible.
Karl Miller; Richann Jean Watson
With the release of the new ADaM Occurrence Data Model for public comment in the first quarter of 2014, the new model is clearly established to encompass adverse events as well as concomitant medications, along with other data into this standard occurrence analysis structure. Commonly used analysis for this type of occurrence structure data can be found utilizing subject counts by category, based on certain criteria (e.g. treatment, cohort, or study period).
In most cases, the majority of the analysis data will be in a one-to-one relationship with the source SDTM record.
In this paper, the authors will discuss the creation of ADaM occurrence data for specific cases outside the common or typical analysis where analysis requires a record in SDTM data, which spans across multiple study treatments, periods or phases, to be replicated for inclusion in non-typical analysis with a record being analyzed under multiple study treatments, periods or phases. From the assignment and imputation of key timing variables (i.e. APERIOD, APHASE, ASTDT), through the appropriate derivation of indicator variables and occurrence flags (i.e. ANLzzFL, TRTEMFL, ONTRTFL and AOCCRFL, AOCCPFL, etc.) the authors guide you through the non-typical process in order to maintain efficiency along with ensuring the traceability in the generation of this analysis-ready data structure.
Richann Jean Watson; Karl Miller
Investigation of drug safety issues for clinical development will consistently revolve around the experience and impact of important medical occurrences throughout the conduct of a clinical trial. As a first step in the data analysis process, Standardized MedDRA Queries (SMQs), a unique feature of MedDRA, provide a consistent and efficient structure to support safety analysis, reporting, and also address important topics for regulatory and industry users. A variance in working with SMQs is the ability to limit the scope for the analysis need (e.g., “Broad” or “Narrow”) but there is also the ability outside of the specific SMQs in allowing the ability to develop Customized Queries (CQs). With the introduction of the ADaM Occurrence Data Structure (OCCDS) standard structure, the incorporation of these SMQs, along with potential CQs, solidified the need for consistent implementation, not only across studies, but across drug compounds and even within a company itself. Working with SMQs one may have numerous questions: What differentiates the SMQ from a CQ and which one should be used? Are there any other considerations in implementation of the OCCDS standards? Where does one begin? Right here…
Richann Jean Watson; Karl Miller
The ADaM Implementation Guide was created in order to help maintain a consistency for the development of analysis data sets in the pharmaceutical industry. However, since its inception we have seen issues with guideline non-conformance which can impede this development process and carry impacts that are felt down-stream in subsequent processes. When working with ADaM data sets, non-compliance and other related issues are likely the number one source for numerous hours of re-work; not only creating unnecessary additional work for the data sets themselves, but also for reports, compliance checks, the Analysis Data Reviewers Guide (ADRG), etc. all the way down to the ISS/ISE processes. Considering this breadth of impact, one can see how devastating these sinkholes can be. Like any sinkhole, there is a way out of it but it is a long, tedious process that will consume a lot of resources and it is always better to avoid the sinkhole entirely. This paper will assist you in creating compliant ADaM data sets, provide the reasoning on why you should avoid these sinkholes, all of which will help minimize re-work and likely eliminate the need for additional work.
Sandra Minjoe; Wayne Zhong; Quan (Jenny) Zhou; Kent Letourneau; Richann Jean Watson
One of the fundamental principles of ADaM is that datasets and associated metadata must include traceability as a link between analysis results, ADaM datasets, and SDTM datasets. The existing ADaM documents contain some examples of simple traceability, such as variable derivations and inclusion of the SDTM sequence number, but what about more complex examples?
An ADaM sub-team is currently developing a Traceability Examples Document, showing how traceability can be employed in a wide variety of practical scenarios. Some of these examples contain content from other CDISC documents, modified to focus on the traceability aspects. Others are being developed specifically for the Traceability Examples Document. As members of the Traceability Examples ADaM sub-team, we are including in this PharmaSUG paper and presentation a selection of examples that demonstrate the power of traceability in complex analyses.
Wayne Zhong, Accretion Softworks; Richann Jean Watson, DataRich Consulting; Daphne Ewing, CSL Behring; Jasmine Zhang, Boehringer Ingelheim
One of the fundamental principles of ADaM is that datasets and associated metadata must include traceability to facilitate the understanding of the relationships between analysis results, ADaM datasets, and SDTM datasets. The existing ADaM documents contain isolated elements of traceability, such as including SDTM sequence numbers, creating new records to capture derived analysis values, and providing excerpts of define.xml documentation.
An ADaM sub-team is currently developing a Traceability Examples Document with the goal of bringing these separate elements of traceability together and demonstrate how they function in detailed and complete examples. The examples cover a wide variety of practical scenarios; some expand on content from other CDISC documents, while others are developed specifically for the Traceability Examples Document. As members of the Traceability Examples ADaM sub-team, we are including in this PharmaSUG paper a selection of examples to show how traceability can bring transparency and clarity to your analyses.
Lindsey Xie, Jinlin Wang, Jennifer Sun and Rita Lai, Kite Pharma, a Gilead Company; Richann Jean Watson, DataRich Consulting
Processing and presenting lab data is always challenging, especially when lab limits are assessed in two directions. The lab data process becomes even more complicated when multiple baselines are required due to different analysis criteria or are inherent in the study design. This paper discusses an approach to create lab toxicity grade variables in ADLB for lab bi-directional toxicity report. They are mixed variables defined by Clinical Data Interchange Standards Consortium (CDISC) Analysis Data Model Implementation Guide (ADaMIG) v1.1 and draft ADAMIG v1.2 and by sponsor to make ADLB easily interpreted and related summary tables easily produced.
This paper is based on the lab Common Terminology Criteria for Adverse Events (CTCAE) toxicity grade summary, taking into account lab tests with abnormal assessment in either increased direction or decreased direction. In this paper, the authors explain and provide examples showing how ADaMIG v1.1 variables ATOXGR, BTOXGR, SHIFTy, ANLzzFL, MCRITy, and BASETYPE, draft ADaMIG v1.2 new variables ATOXGRH(L) and BTOXGRH(L), and sponsor-defined variables ATOXDIR and WAYSHIFT can be utilized and implemented appropriately. In addition, this paper explains how to handle baseline toxicity grade for analysis sets with more than one baseline in ADLB.
Lindsey Xie, Jinlin Wang, Lauren Xiao, Kite Pharma, Inc.; Richann Jean Watson
Due to the various data needed for safety occurrence analyses, the use of a child data set that contains all the data for a given data point aids in traceability and support of the analysis.
Adverse Events of Special Interest (AESI) represents adverse events (AEs) that are of particular interest in the study. These AESI could potentially have symptoms associated with them. AESI could be captured as clinical events (CEs) in the CE domain while the associated symptoms for each CE are captured as AEs in the AE domain.
The relationship between CEs and associated AE symptoms are an important part of the safety profile for a compound in clinical trials. These relationships are not always readily evident in the source data or in a typical AE analysis data set (ADAE). The use of a child data set can help demonstrate this relationship, which provides enhanced data traceability to help the review effort for both sponsor and regulatory agency reviewers.
This paper provides examples of using a child data set to preserve data with the relationship between CEs and associated AE symptoms in an ADAE child data set (ADAE<si>). In addition, the paper will show that ADAE and ADAE<si> serve as analysis-ready data sets for the summary of AE and AESI.
Richann Jean Watson; Karl Miller
Ordinarily, Standardised MedDRA Queries (SMQs) aim is to group specific MedDRA terms for a defined medical condition or area of interest at the Preferred Term (PT) level, which most would consider to be the basic use of SMQs. However, what if your study looks to implement the use of SMQs that goes beyond the basic use? Whether grouping through the use of algorithmic searching, using weighted terms or not, or through the use of hierarchical relationships, this paper looks to cover advanced searches that will take you beyond the basics of working with SMQs. Gaining insight to this process will help you become more familiar in working with all types of SMQs and will put you in a position to become the "go-to" person for helping others within your company.
Kaleigh Ragan, Crinetics Pharmaceuticals; Richann Jean Watson
The Related Records (RELREC) special purpose dataset is a tool, provided in the Study Data Tabulation Model (SDTM), for conveying relationships between records housed in different domains. Most SDTM users are familiar with a one-to-one relationship type, where a single record from one domain is related to a single record in a separate domain. Or even a one-to-many relationship type, where a single record from one domain may be related to a group of records in another. But what if there are two groups of records related to one another? How do you properly convey the relationship between these sets of data points? This paper aims to provide a clearer understanding of when and how to utilize the, not often encountered, many-to-many relationship type within the RELREC special purpose dataset.
Richann Jean Watson; Elizabeth Dennis, EMB Statistical Solutions LLC; Karl Miller, IQVIA
For analysis purposes, dataset records are often assigned to an analysis timepoint window rather than simply using the visits or timepoints from the collected data. The rules for analysis timepoint windows are usually defined in the Statistical Analysis Plan (SAP) and can involve complicated derivations to determine which record(s) best fulfils the analysis window requirements. For traceability, there are ADaM standard variables available to help explain how records are assigned to the analysis windows. This paper will explore these ADaM variables and provide examples on how they may be applied.
Inka Leprince, PharmaStat LLC, Richann Jean Watson
In the intricate dance of clinical trials that involve multiple treatment groups and varying dose levels, subjects pirouette through planned treatments - each step assigned with precision. Yet, in the realms of pediatric, oncology, and diabetic trials, the challenge arises when planned doses twirl in the delicate arms of weight-adjustments. How can data analysts choreograph the Analysis Data Model (ADaM) data sets to capture these nuanced doses?
There is a yearning to continue with the normal dance routine of analyzing subjects based on their protocol-specified treatments, yet at times it is necessary to learn a new dance step, so as not to overlook the weight-adjusted doses the subjects actually received. The treatment variables TRTxxP/N in the Subject-Level Analysis Dataset (ADSL) and their partners TRTP/N in Basic Data Structure (BDS) and Occurrence Data Structure (OCCDS) are elegantly designed to ensure each treatment glides into its designated column in the summary tables. But we also need to preserve the weight-adjusted dose level on a subject- and record-level basis. DOSExxP and DOSExxA, gracefully twirl in the ADSL arena, while their counterparts, the dashing DOSEP and DOSEA, lead the waltz in the BDS and OCCDS data sets. Together, these harmonious variables pirouette across the ADaM data sets, capturing the very essence of the weight-adjusted doses in a dance that seamlessly unfolds.
SAS PAPERS
Richann Jean Watson
Have you ever wanted to calculate the confidence intervals for treatment differences or calculate the least square means using a mixed model but can’t always recall the correct options or layout of the model for PROC MIXED? If so, the macro presented in this paper will appeal to you. The DOMIXED macro allows for the calculation of least square means, standard error, observed mean, standard deviation and confidence intervals for treatment difference. The macro will also calculate p-values.
Richann Jean Watson; Patty Johnson
Validation is an essential part of the derived data set, summary table and listing development process. Validation can require that an independent program be created that confirms the results of the original program are accurate. It is common practice that derived data sets are validated using a more automated method, but it is not as common that table and listing outputs are validated using an automated method. A validation programmer often manually compares the results of her independent programming to the original production output or results. This entails checking not only the titles and footnotes and other cosmetic aspects of the output but also the informational aspect of the output. The manual method of checking the informational aspects of the output is a time consuming process and is subject to human error. This paper presents an automated approach for performing a 100% validation of the informational portion of summary table and listing outputs.
Richann Jean Watson
Creating visit windows is sometimes required for analysis of data. We need to make sure that we get the visit/day in the proper window so that the data can be analyzed properly. However, defining these visit windows can be quite cumbersome especially if they have to be defined in numerous programs. This task can be made easier by applying a picture format, which can save a lot of time and coding. A format is easier to maintain than a bunch of individual programs. If a change to the algorithm is required, the format can be updated instead of updating all of the individual programs containing the visit definition code.
Richann Jean Watson
Making sure that you have saved all the necessary information to replicate a deliverable can be a cumbersome task. You want to make sure that all the raw data sets and all the derived data sets, whether they are Study Data Tabulation Model (SDTM) data sets or Analysis Data Model (ADaM) data sets, are saved. You prefer that the date/time stamps are preserved. Not only do you need the data sets, you also need to keep a copy of all programs that were used to produce the deliverable, as well as the corresponding logs from when the programs were executed. Any other information that was needed to produce the necessary outputs also needs to be saved. You must do all of this for each deliverable, and it can be easy to overlook a step or some key information. Most people do this process manually. It can be a time-consuming process, so why not let SAS® do the work for you?
Richann Jean Watson; Karl Miller
There are times when we need to use the attributes of a variable within a data set. Normally, this can be done with a simple CONTENTS procedure. The information can be viewed prior to programming and then hardcoded within the program or it can be saved to a data set that can be joined back to the main data set. If the attributes are hardcoded then what happens if the data set changes structure, then the program would need to be updated accordingly. If the information from PROC CONTENTS is saved and then joined with the main data set, then this would need to be done for all data sets that need to be processed. This is where knowing your ‘V’ functions can come in handy. The ‘V’ functions can be used to return the label, format, length, name, type and/or value of a variable or a string within the data step. These functions can come quite in handy when you need to create summary statistics and if you need to perform an algorithm on a variable with a specific naming convention.
Richann Jean Watson; Lynn Mullins, PPD
There are often times when programmers need to merge multiple SAS® data sets to combine data into one single source data set. Like many other processes, there are various techniques to accomplish this using SAS software. The most efficient method to use based on varying assumptions will be explored in this paper. We will describe the differences, advantages and disadvantages, and display benchmarks of using HASH tables, the SORT and DATA step procedures, and the SQL procedure.
Richann Jean Watson
In the pharmaceutical industry, we find ourselves having to re-run our programs repeatedly for each deliverable. These programs can be run individually in an interactive SAS® session, which enables us to review the logs as we execute the programs. We could run the individual programs in batch and open each individual log to review for unwanted log messages, such as ERROR, WARNING, uninitialized, have been converted to, and so on. Both of these approaches are fine if there are only a handful of programs to execute. But what do you do if you have hundreds of programs that need to be re-run? Do you want to open every single one of the programs and search for unwanted messages? This manual approach could take hours and is prone to accidental oversight. This paper discusses a macro that searches a specified directory and checks either all the logs in the directory, only logs with a specific naming convention, or only the files listed. The macro then produces a report that lists all the files checked and indicates whether issues were found.
Richann Jean Watson; Karl Miller
Have you ever been working on a task and wondered whether there might be a SAS® function that could save you some time? Let alone, one that might be able to do the work for you? Data review and validation tasks can be time-consuming efforts. Any gain in efficiency is highly beneficial, especially if you can achieve a standard level where the data itself can drive parts of the process. The ANY and NOT functions can help alleviate some of the manual work in many tasks such as data review of variable values, data compliance, data formats, and derivation or validation of a variable's data type. The list goes on. In this poster, we cover the functions and their details and use them in an example of handling date and time data and mapping it to ISO 8601 date and time formats.
Richann Jean Watson; Lynn Mullins, PPD
As programmers, we are often asked to program statistical analysis procedures to run against the data. Sometimes the specifications we are given by the statisticians outline which statistical procedures to run. But other times, the statistical procedures to use need to be data dependent. To run these procedures based on the results of previous procedures' output requires a little more preplanning and programming. We present a macro that dynamically determines which statistical procedure to run based on previous procedure output. The user can specify parameters (for example, fshchi, plttwo, catrnd, bimain, and bicomp), and the macro returns counts, percents, and the appropriate p-value for Chi-Square versus Fisher Exact, and the p-value for Trend and Binomial CI, if applicable.
Richann Jean Watson; Joshua Horstman, Nested Loop Consulting
Validation of analysis datasets and statistical outputs (tables, listings, and figures) for clinical trials is frequently performed by double programming. Part of the validation process involves comparing the results of the two programming efforts. COMPARE procedure output must be carefully reviewed for various problems, some of which can be fairly subtle. In addition, the program logs must be scanned for various errors, warnings, notes, and other information that might render the results suspect. All of this must be performed repeatedly each time the data is refreshed or a specification is changed. In this paper, we describe a complete, end-to-end, automated approach to the entire process that can improve both efficiency and effectiveness.
Kriss Harris, SAS Specialists Limited; Richann Jean Watson
When reporting your safety data, do you ever feel sorry for the person who has to read all the laboratory listings and summaries? Or have you ever wondered if there is a better way to visualize safety data? Let’s use animation to help the reviewer and to reveal patterns in your safety data, or in any data!
This hands-on workshop demonstrates how you can use animation in SAS® 9.4 to report your safety data, using techniques such as visualizing a patient’s laboratory results, vital sign results, and electrocardiogram results and seeing how those safety results change over time. In addition, you will learn how to animate adverse events over time, and how to show the relationships between adverse events and laboratory results using animation. You will also learn how to use the EXPAND procedure to ensure that your animations are smooth. Animating your data will bring your data to life and help improve lives!
Kriss Harris, SAS Specialists Limited; Richann Jean Watson
It’s a Great Time to Learn GTL! Do you want to be more confident when producing GTL graphs? Do you want to know how to layer your graphs using the OVERLAY layout and build upon your graphs using multiple LAYOUT statement? This paper guides you through the GTL fundamentals!
Richann Jean Watson; Louise Hadden, Independent Consultant.
SAS® practitioners are frequently called upon to do a comparison of data between two different data sets and find that the values in synonymous fields do not line up exactly. A second quandary occurs when there is one data source to search for particular values, but those values are contained in character fields in which the values can be represented in myriad different ways. This paper discusses robust, if not warm and fuzzy, techniques for comparing data between, and selecting data in, SAS data sets in not so ideal conditions.
Kriss Harris, SAS Specialists Limited; Richann Jean Watson
This paper demonstrates how you can use interactive graphics in SAS® 9.4 to assess and report your safety data. The interactive visualizations that you will be shown include the adverse event and laboratory results. In addition, you will be shown how to display "details-on-demand" when you hover over a point.
Adding interactivity to your graphs will bring your data to life and help improve lives!
Richann Jean Watson
Output Delivery System (ODS) graphics, produced by SAS® procedures, are the backbone of the Graph Template Language (GTL). Procedures such as the Statistical Graphics (SG) procedures dynamically generate GTL templates based on the plot requests made through the procedure syntax. For this paper, these templates will be referenced as procedure-driven templates. GTL generates graphs using a template definition that provides extensive control over output formats and appearance. Would you like to learn how to build your own template and make customized graphs and how to create that one highly desired, unique graph that at first glance seems impossible? Then it’s a Great Time to Learn GTL! This paper guides you through the GTL fundamentals while walking you through creating a graph that at first glance appears too complex but is truly simple once you understand how to build your own template.
Richann Jean Watson; Louise Hadden, Independent Consultant
A typical task for a SAS® practitioner is the creation of a new variable that is based on the value of another variable or string. This task is frequently accomplished by the use of IF-THEN-ELSE statements. However, manually typing a series of IF-THEN-ELSE statements can be time-consuming and tedious, as well as prone to typos or cut and paste errors. Serendipitously, SAS has provided us with an easier way to assign values to a new variable. The WHICH and CHOOSE functions provide a convenient and efficient method for data-driven variable creation.
Richann Jean Watson
The appearance of a graph produced by the Graph Template Language (GTL) is controlled by Output Delivery System (ODS) style elements. These elements include fonts and line and marker properties as well as colors. A number of procedures, including the Statistical Graphics (SG) procedures, produce graphics using a specific ODS style template. This paper provides a very basic background of the different style templates and the elements associated with the style templates. However, sometimes the default style associated with a particular destination does not produce the desired appearance. Instead of using the default style, you can control which style is used by indicating the desired style on the ODS destination statement. However, sometimes not a single one of the 50-plus styles provided by SAS® achieves the desired look. Luckily, you can modify an ODS style template to meet your own needs. One such style modification is to control which colors are used in the graph. Different approaches to modifying a style template to specify colors used are discussed in depth in this paper.
Richann Jean Watson; Louise Hadden, Independent Consultant
Let’s admit it! We have all been on a conference call that just … well to be honest, it was just bad. Your misery could be caused by any number of reasons – or multiple reasons! The audio quality was bad, the conversation got sidetracked and focus of the meeting was no longer what it was intended, there could have been too much background noise, someone hasn’t muted their laptop and is breathing heavily – the list goes on ad nauseum. Regardless of why the conference call is less than satisfactory, you want it to end, but professional etiquette demands that you remain on the call. We have the answer – SAS®-generated Conference Call Bingo! Not only is Conference Call Bingo entertaining, but it also keeps you focused on the conversation and enables you to obtain the pertinent information the conference call may offer.
This paper and presentation introduce a method of using SAS to create custom Conference Call Bingo cards, moving through brainstorming and collecting entries for Bingo cards, random selection of items, and the production of bingo cards using SAS reporting techniques and the Graphic Template Language (GTL). (You are on your own for the chips and additional entries based on your own painful experiences)! The information presented is appropriate for all levels of SAS programming and all industries.
Richann Jean Watson; Louise Hadden, Independent Consultant
SAS® Functions have deservedly been the focus of many excellent SAS papers. SAS CALL subroutines, which rely on and collaborate with SAS functions, are less well known, although many SAS programmers use these subroutines frequently. This paper will look at numerous SAS functions and CALL subroutines, as well as explaining how both SAS functions and CALL subroutines work in practice.
There are many areas that SAS CALL subroutines cover including CAS (Cloud Analytic Services) specific functions, character functions, character string matching, combinatorial functions, date and time functions, external subroutines, macro functions, mathematical functions, sort functions, random number functions, special functions, variable control functions, and variable information functions.
While there are myriad SAS CALL subroutines and SAS functions, we plan to drill down on character function SAS CALL subroutines including string matching; macro, external and special subroutines; sort subroutines; random number generation subroutines; and variable control and information subroutines. We could go on and on about SAS CALL subroutines, but we are going to limit the SAS CALL subroutines discussed in this paper, excluding any environment specific SAS CALL subroutines such as those designated for use with CAS and TSO, as well as other redundant examples. We hope to demystify SAS CALL subroutines by demonstrating real world applications of specific SAS CALL subroutines, bringing some amazing capabilities to light.
Richann Jean Watson
Programmers frequently have to deal with dates and date formats. At times, determining whether a date is in a day-month or month-day format can leave us confounded. Clinical Data Interchange Standards Consortium (CDISC) has implemented the use of the International Organization for Standardization (ISO) format, ISO® 8601, for datetimes in SDTM domains, to alleviate the confusion. However, converting “datetimes” from the raw data source to the ISO 8601 format is no picnic. While SAS® has many different functions and CALL subroutines, there is not a single magic function to take raw datetimes and convert them to ISO 8601. Fortunately, SAS allows us to create our own custom functions and subroutines. This paper illustrates the process of building a custom function with custom subroutines that takes raw datetimes in various states of completeness and converts them to the proper ISO 8601 format.
Richann Jean Watson; Louise Hadden, Independent Consultant.; Deanna Schreiber-Gregory
SAS functions act as subroutines, combining arguments, operations and optional parameters, and they return a value as a result. These values can be either numeric or characters and can be used to create variables in expressions, via the DATA step, WHERE statements, the SAS macro language, within PROC REPORT and in PROC SQL.
CALL routines are very similar to SAS functions, sometimes sharing the same name and providing the same or similar functionality. CALL routines, as the name implies, begin with CALL statements, with the routine name appearing after “CALL”, followed by parenthetical arguments. As with SAS functions, the number of required and optional arguments vary.
Functions and CALL routines fall into specific categories, including character and string-matching functions and CALL routines and mathematical functions. Our favorite functions, PRXMATCH and PRXCHANGE, CATT and CATX and mathematical functions, fall into these two broad categories. We will provide descriptions of our favorite functions, guidance on how to use these functions and individual business use examples.
There is no paper associated with this topic, only a live recording.
Lisa Mendez, Catalyst Clinical Research; Richann Jean Watson
There are many useful tips that do not warrant a full paper but put some cool SAS® code together and you get a cocktail of SAS code goodness. This paper will provide ten great coding techniques that will help enhance your SAS programs. We will show you how to 1) add quotes around each tokens in a text string, 2) create column headers based values using Transpose Procedure (PROC TRANSPOSE), 3) set missing values to zero without using a bunch of if-then-else statements, 4) using short-hand techniques for assigning lengths of consecutive variables and for initializing to missing, 5) calculating the difference between the visit's actual study day and the target study and accounting for no day zero (0); 6) use SQL Procedure (PROC SQL) to read variables from a data set into a macro variable, 7) use the XLSX engine to read data from multiple Microsoft® Excel® Worksheets, 8) test to see if a file exists before trying to open it, such as an Microsoft Excel file, 9) using the DIV function to divide so you don’t have to check if the value is zero, and 10) use abbreviations for frequently used SAS code.
Kirk Paul Lafler, sasNerd; Richann Jean Watson; Joshua Horstman, Nested Loop Consulting; Charu Shankar, SAS Institute Inc.
Should I use the DATA step or PROC SQL to process my data? Which approach will give me the control, flexibility, and scale to process data exactly the way I want it? Which approach is easier to use? Which approach offers the greatest power and capabilities? And which approach is better? If you have these and other questions about the pros and cons of the DATA step versus PROC SQL, this presentation is for you. We will discuss, using real-life scenarios, the strengths (and even a few weaknesses) of the two most powerful and widely used data processing approaches in SAS® (as we see it). We will provide you with the knowledge you need to make that difficult decision about which approach to use to process all that data you have.
Joshua Horstman, Nested Loop Consulting; Richann Jean Watson
The SAS macro facility is an amazing tool for creating dynamic, flexible, reusable programs that automatically adapt to change. This presentation uses examples to demonstrate how to transform static "muggle" code full of hardcodes and data dependencies by adding macro language magic to create data-driven programming logic. Cast a vanishing spell on data dependencies and let the macro facility write your SAS code for you!
Richann Jean Watson; Louise Hadden, Independent Consultant.
SAS® provides a vast number of functions and subroutines (sometimes referred to as CALL routines). These useful scripts are an integral part of the programmer’s toolbox, regardless of the programming language. Sometimes, however, pre-written functions are not a perfect match for what needs to be done, or for the platform that required work is being performed upon. Luckily, SAS has provided a solution in the form of the FCMP procedure, which allows SAS practitioners to design and execute User-Defined Functions (UDFs). This paper presents two case studies for which the character or string functions SAS provides were insufficient for work requirements and goals and demonstrate the design process for custom functions and how to achieve the desired results.
Lisa Mendez, Catalyst Clinical Research; Richann Jean Watson
This paper provides an overview of six SAS CALL subroutines that are frequently used by SAS® programmers but are less well-known than SAS functions. The six CALL subroutines are CALL MISSING, CALL SYMPUTX, CALL SCAN, CALL SORTC/SORTN, CALL PRXCHANGE, and CALL EXECUTE.
Instead of using multiple IF-THEN statements, the CALL MISSING subroutine can be used to quickly set multiple variables of various data types to missing. CALL SYMPUTX creates a macro variable that is either local or global in scope. CALL SCAN looks for the nth word in a string. CALL SORTC/SORTN is used to sort a list of values within a variable. CALL PRXCHANGE can redact text, and CALL EXECUTE lets SAS write your code based on the data.
This paper will explain how those six CALL subroutines work in practice and how they can be used to improve your SAS programming skills.
Richann Jean Watson; Joshua Horstman, Nested Loop Consulting
The ODS Statistical Graphics package is a powerful tool for creating the complex, highly customized graphs often produced when reporting clinical trial results. These tools include the ODS Statistical Graphics procedures, such as the SGPLOT procedure, as well as the Graph Template Language (GTL). The SG procedures give the programmer a convenient procedural interface to much of the functionality of ODS Statistical Graphics, while GTL provides unparalleled flexibility to customize nearly any graph that one can imagine. In this hands-on workshop, we step through a series of increasingly sophisticated examples demonstrating how to use these tools to build clinical graphics by starting with a basic plot and adding additional elements until the desired result is achieved.
Richann Jean Watson
Within the life sciences, programmers often find themselves doing a lot of dating – matching, converting between character and numeric values, and imputing missing components. Clinical Data Interchange Standards Consortium (CDISC) Study Data Tabulation Model (SDTM) domains have implemented the use of the International Organization for Standardization (ISO) format, ISO® 8601, for datetimes. These dates are stored as character strings with missing components denoted using a single hyphen. Although this format helps to standardize how dates and times are captured so that there is no confusion as to what the date represents, it leaves a longing for something more compatible for analysis purposes: determining durations and number of days from a reference point. The conversion of ISO dates to a numeric format requires a serious commitment, especially when partial ISO dates require imputations. Although SAS® offers a variety of built-in formats and functions that can appease both sides on a date, i.e., converting complete ISO dates to numeric values or numeric dates to ISO dates, there is no SAS-provided function that will help with the required conversion and imputation of a partial date. Fortunately, with the use of the FCMP procedure, we can create our own custom functions to help achieve our desired goal. This paper illustrates the process of building a custom function that will take a date that is captured in the appropriate ISO format in SDTM (--DTC) and convert that date to a numeric format while also giving partial dates the extra attention to impute missing components. Additionally, this custom function sets the correct date imputation variable (--DTF), so you always know just how much of a blind date your derived value really is.
Joshua J. Cook, University of West Florida; Richann Jean Watson
The transition from SDTM to ADaM data sets is a critical step in clinical trial data preparation, requiring precise programming solutions to ensure compliance with CDISC standards. This paper presents a two-stage approach to ADSL derivation: first, we manually derive key variables using base R and the tidyverse, demonstrating the detailed logic required for compliance and flexibility in handling real-world data challenges. Then, we explore the admiral package, showcasing how its specialized functions significantly simplify and streamline this process. By leveraging admiral, we automate derivations such as treatment start and end dates (TRTSDT, TRTEDT) and treatment duration (TRTDUR) while addressing duplicates, validating data set integrity, and enhancing reproducibility. We highlight the power of admiral in minimizing code redundancy, integrating custom logic, and ensuring seamless compliance with industry standards. To aid in the transition of code from SAS® to R, this paper includes a SAS version of the code for comparison purposes. Designed for intermediate to advanced programmers, this paper illustrates the benefits of transitioning to open-source tools and the pharmaverse ecosystem for robust clinical data workflows.
Lisa Mendez, Army MEDCOM; Richann Jean Watson
You've spent hours crafting your SAS® code, or perhaps you've inherited a jumbled mess from someone else, and when you run it, it "explodes" like an ill-fated cocktail experiment and leaves a bad taste in your mouth! Mastering the art of debugging not only makes coding a smoother experience but also spares you from needing a stiff drink to cope with the frustration.
This paper will discuss tips, tricks, and techniques for debugging your SAS code. It will show you how to use the debugger in both SAS Display Manager and SAS Enterprise Guide, akin to a bartender knowing just the right tools to mix the perfect drink. It will also describe how to use PUTLOG and PUT statements, along with their key differences—like choosing between a whiskey neat and a whiskey sour.
Additional debugging aids such as using OPTIONS, and macro variables will be discussed, ensuring you have a well-stocked bar of techniques at your disposal. As a bonus, the paper will also provide some common error messages and their meanings—your personal cocktail menu of debugging solutions.
Joshua Horstman, PharmaStat; Richann Jean Watson
In their popular 2024 presentation "From Muggles to Macros", Horstman and Watson delivered a spell book full of macro magic to enhance SAS programs with data-driven wizardry. This exciting sequel to that enchanting performance adds to the list of incantations for creating dynamic, flexible, reusable programs that can automatically adapt to change. New charms include the use of control tables, the CALL EXECUTE routine, and of course, more macro language techniques. Don't hardcode data values into your programs. Cast a vanishing spell on data dependencies and let the macro facility write your SAS code for you!
Joshua Horstman, PharmaStat; Richann Jean Watson
Patient profiles are often used to monitor the conduct of a clinical trial, detect safety signals, identify data entry errors, and catch protocol deviations. Each profile combines key data collected regarding a single subject – everything from dosing to adverse events to lab results. In this presentation, two experienced statistical programmers share how to leverage the SAS Macro Language, Output Delivery System (ODS), the REPORT procedure, and ODS Statistical Graphics to blend both tabular and graphical elements. The result is beautiful, highly-customized, information-rich patient profiles that meet the requirements for managing a modern clinical trial.
MISCELLANEOUS PAPERS
Richann Jean Watson; Louise Hadden, Independent Consultant
Whether you are a first-time conference attendee or an experienced conference attendee, this paper can help you in getting the most out of your conference experience. As long-standing conference attendees and volunteers, we have found that there are some things that people just don’t think about when planning their conference attendance. In this paper we will discuss helpful tips such as making the appropriate travel arrangements, what to bring, networking and meeting up with friends and colleagues, and how to prepare for your role at the conference. We will also discuss maintaining a workplace presence with your paying job while at the conference.
Note this paper has been updated to incorporate the virtual component. The updated paper is titled "Are you Ready? Preparing and Planning to Make the Most of your Conference Experience In Person, Virtual and Hybrid".
Richann Jean Watson; Louise Hadden, Independent Consultant
Whether you are a first-time conference attendee or an experienced conference attendee, this paper can help you in getting the most out of your conference experience. As long-standing conference attendees and volunteers, we have found that there are some things that people just don’t think about when planning their conference attendance. In this paper we will discuss helpful tips such as making the appropriate travel arrangements for in-person and hybrid conferences, setting up your home office space for a virtual conference, what to bring and/or have on hand, networking and meeting up with friends and colleagues both in person and virtually, and how to prepare for your role at the conference, whatever the format. We will also discuss maintaining a workplace presence with your paying job while present in any form at the conference.
Joshua Horstman, Nested Loop Consulting; Richann Jean Watson
While many statisticians and programmers are content in a traditional employment setting, others yearn for the freedom and flexibility that come with being an independent consultant. In this paper, two seasoned consultants share their experiences going independent. Topics include the advantages and disadvantages of independent consulting, getting started, finding work, operating your business, and what it takes to succeed. Whether you're thinking of declaring your own independence or just interested in hearing stories from the trenches, you're sure to gain a new perspective on this exciting adventure.
Richann Jean Watson; Louise Hadden, Independent Consultant
Simplifying and streamlining workflows is a common goal of most programmers. The most powerful and efficient solutions may require practitioners to step outside of normal operating procedures and outside of their comfort zone. Programmers need to be open to finding new (or old) techniques to achieve efficiency and elegance in their code: SAS® by itself may not provide the best solutions for such challenges as ensuring that batch submits preserve appropriate log and lst files; documenting and archiving projects and folders; and unzipping files programmatically. In order to adhere to such goals as efficiency and portability, there may be times when it is necessary to utilize other resources, especially if colleagues may need to perform these tasks without the use of SAS software. These and other data management tasks may be performed via the use of tools such as command-line interpreters and Windows PowerShell (if available to users), used externally and within SAS software sessions. We will also discuss the use of additional tools, such as WinZip®, used in conjunction with the Windows command-line interpreter.